How swarming animals can help humans and AI make better decisions
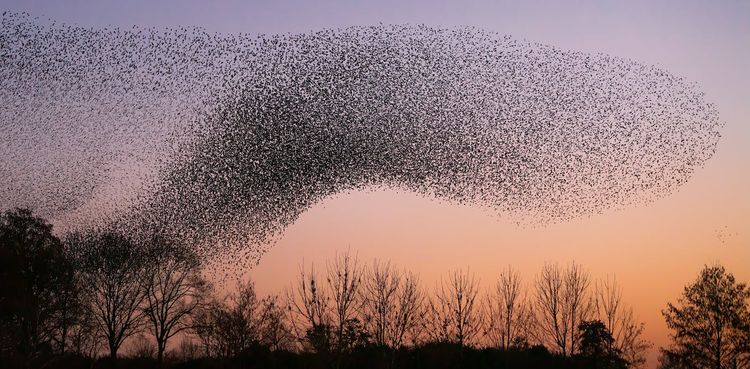
The term swarm commonly has unfavorable implications – envision uncontrollable masses of locusts as mentioned in the Bible or bustling streets filled with last-minute shoppers during the Christmas frenzy. Nevertheless, swarming plays a vital role in ensuring the endurance of various animal groups. Additionally, studies on swarming hold the promise of revolutionizing human endeavors as well.
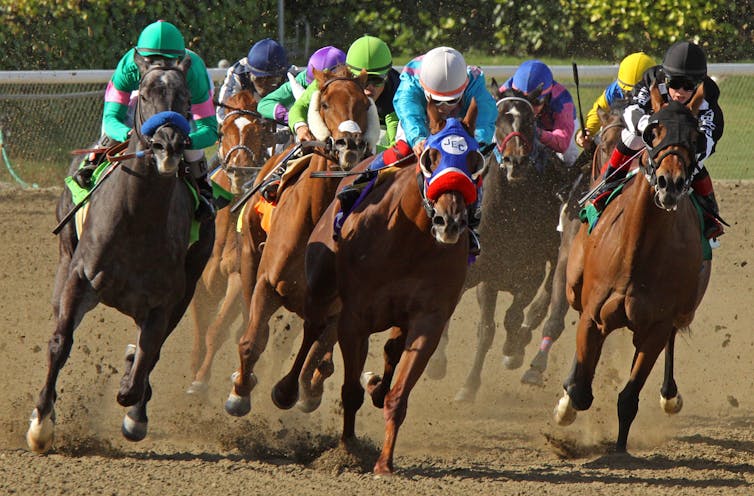
Bees gather together in large groups to improve their efficiency in finding new homes. Groups of starlings create mesmerizing patterns while flying to escape and puzzle their predators. These are only a couple of instances observed in the natural world, but swarming behavior can be observed across different animal species and ecosystems.
Studies conducted by individuals in the fields of mathematics, biology, and social science are providing valuable insights into the phenomenon of swarming and enabling us to utilize its potential. Currently, swarming tactics are already being implemented for purposes such as managing crowds, controlling traffic, and comprehending the transmission of contagious diseases. Furthermore, it is increasingly influencing the healthcare industry's utilization of data, the deployment of military drones in conflict zones, and even aiding in achieving improbable victories in sports betting scenarios.
A collective is a structure that is more powerful when combined than when considered separately. Similar to how numerous neurons come together to create a mind capable of thinking, remembering, and feeling, clusters of creatures can collaborate to build a "super mind", showcasing extremely intricate conduct absent in solitary beings.
In 1986, Craig Reynolds, an expert in artificial life, completely transformed the way we understand and study the behavior of swarms through his groundbreaking computer simulation known as the Boids model. This innovative model effectively deconstructs the complex phenomenon of swarming into a basic set of rules.
The Boids (bird-oids) in the simulation, similar to avatars or characters in a video game, are guided to travel alongside their nearby companions, head towards the middle spot of their companions, and steer clear of any clashes with fellow boids.
Boids simulations show remarkable precision when compared to actual swarms.
The Boids concept proposes that swarming can occur without the requirement of leaders directing the behavior, similar to how people walk in a city center instead of being guided on a tour at a museum. The intricate actions displayed by swarms are a result of individuals interacting with one another while following basic rules simultaneously. In scientific terms, this phenomenon is referred to as emergence.
In the year 2016, the US-based tech company known as Unanimous AI utilized the strength of swarm intelligence to emerge victorious in betting on the Kentucky Derby "superfecta". Their predictions turned out to be accurate, as they correctly anticipated the ranking of the first, second, third, and fourth riders in this renowned horse race that takes place in the United States.
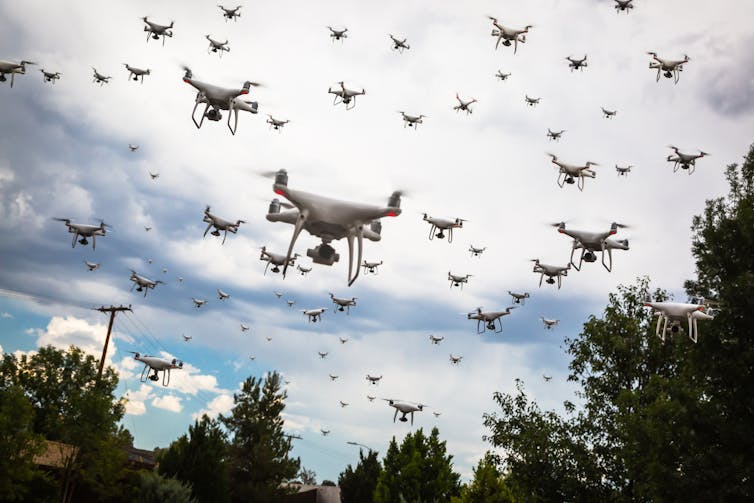
Experts in the field and traditional algorithms for machine learning made a lot of wrong forecasts. Nonetheless, Unanimous AI managed to gather the expertise of racing hobbyists in order to overcome the incredibly low chance of 541/1.
The achievement of the volunteers was attributed to the method through which their forecasts were formed. Instead of casting votes on riders and combining their selections, the volunteers opted for Unanimous AI's swarm intelligence system to engage in a dynamic online battle, drawing inspiration from flocks of birds and colonies of bees.
Every volunteer pulled a knob at the same time, adjusting their preferences based on the choices made by others. For instance, if they noticed that options A and B were becoming the top picks, someone might have switched from their initial choice, C, to pulling towards their second choice, B.
When Unanimous AI's volunteers communicated with each other instantly, they were able to surpass the performance of well-informed individuals working alone.
Moreover, if the volunteers' most commonly chosen selections had determined the sequence, only Nyquist, who won in 2016 and was favored by bookies, would have been positioned accurately.
Comparable clustering technologies are also gaining attention in the healthcare industry, where conversations regarding an AI uprising are causing growing worries regarding the confidentiality of patient information.
As the dependence on data-based methods in the field of healthcare grows, the need for large volumes of patient data also rises. To fulfill these requirements, a possible approach is to combine information from various institutions and, in certain situations, even across borders.
However, the migration of patient information frequently faces rigorous regulations concerning data protection. One potential remedy for this issue involves solely utilizing data that remains within the organization. Nevertheless, this approach often sacrifices the precision of diagnoses.
Another option is the concept of swarming. Scientists are convinced that swarm intelligence has the potential to maintain the accuracy of diagnoses without requiring the direct sharing of raw data among different organizations.
Initial research has indicated that dispersing data storage among a network of interconnected nodes can offer institutions the advantage of collective knowledge. This implies that there is no central hub overseeing the exchange of information, and institutions are unable to retrieve one another's confidential patient data.
In centralized machine learning, data is sent to a central hub where all the machine learning happens using the combined data. On the other hand, decentralized systems have each institution storing its data separately in its own node. Machine learning occurs locally at each node, using only the data from that particular institution. However, the results from the machine learning process are shared between all the nodes in the network, which benefits everyone involved. This process ensures that sensitive patient information is not shared between institutions, thereby protecting patient privacy.
The utilization of drone technology is on the rise in direct confrontations, especially witnessed in the current Russia-Ukraine conflict. Nonetheless, the current state of conventional drone technology necessitates constant individual monitoring.
Present-day military research endeavors to enable communication among unmanned aerial vehicles (UAVs), enabling a single operator to command multiple drone swarms. The emergence of this technology holds great potential in enhancing the expandability, surveillance, and combat prowess of drones by facilitating seamless information sharing within a cluster of these aerial devices.
As studies delve further into the concept of swarming, we discover a realm where combined efforts give rise to intricacy, versatility, and effectiveness. With the advancement of technology, the significance of swarm intelligence is bound to escalate, entwining our reality with the captivating intricacies of swarms.